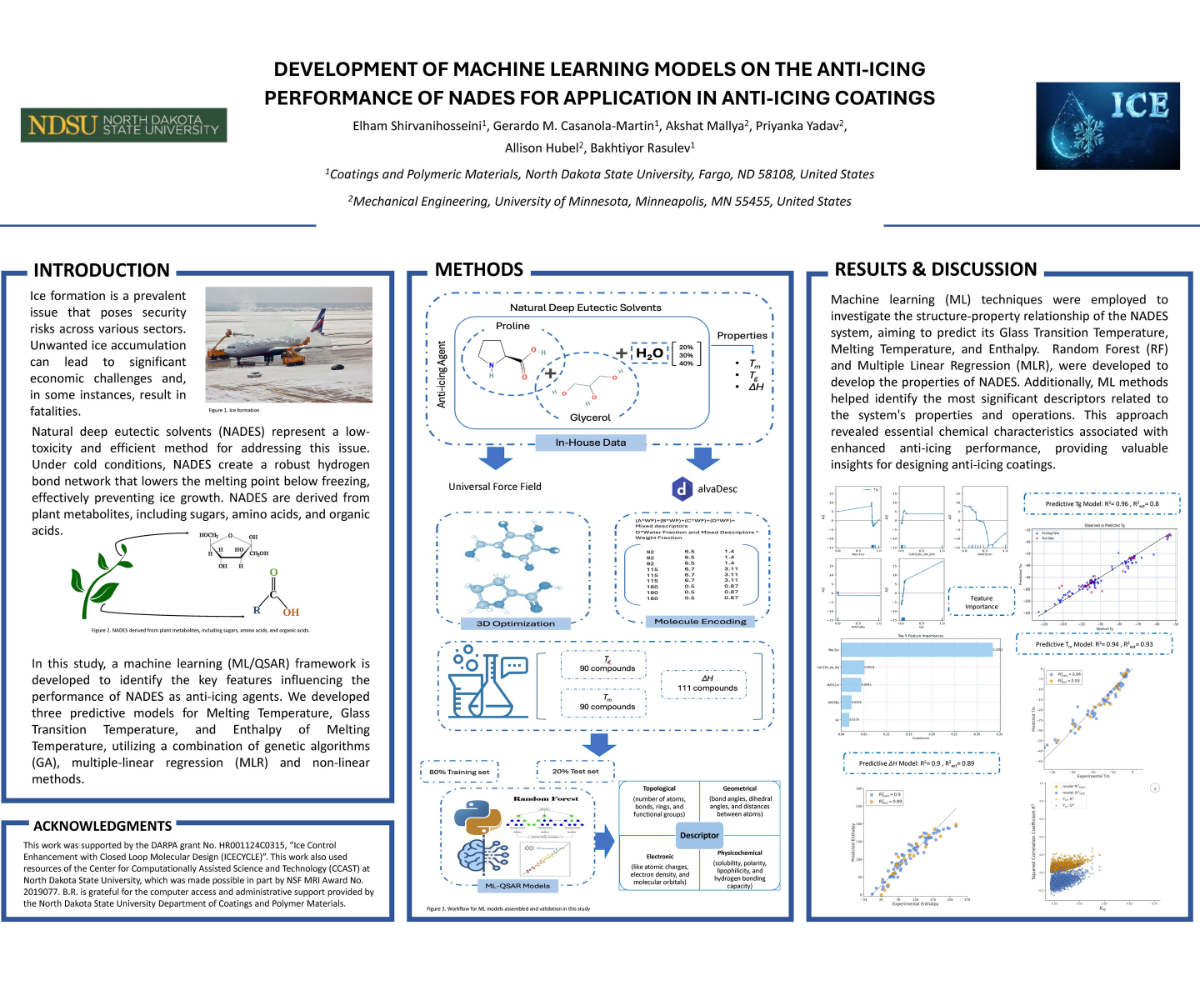
Ice formation remains a critical challenge across multiple industries, posing safety risks, economic burdens, and, in extreme cases, fatalities. Effective anti-icing strategies are essential to mitigate these issues, yet the demand for environmentally friendly, cost-effective, and efficient solutions persists. Natural deep eutectic solvents (NADES) have emerged as a promising low-toxicity alternative for addressing ice formation. Derived from plant metabolites such as sugars, amino acids, and organic acids, NADES form a robust hydrogen bond network under cold conditions, lowering the melting point below freezing and effectively inhibiting ice nucleation and growth. Their versatility has already been demonstrated in industries such as pharmaceuticals, food, cosmetics, and aviation.
In this study, we expanded our investigation into the anti-icing potential of NADES by incorporating additional datasets to enhance the robustness and generalizability of our machine learning (ML/QSAR) framework. Using a combination of genetic algorithm (GA), multiple-linear regression (MLR), and non-linear methods, we developed predictive models for Melting Temperature, Glass Transition Temperature, and Enthalpy of Melting. The inclusion of diverse datasets significantly improved the performance and applicability of the models, resulting in higher predictive accuracy and stronger correlation coefficients. Furthermore, the expanded analysis revealed new insights into the key molecular features influencing NADES performance as anti-icing agents. These findings not only validate the potential of NADES for anti-icing applications but also provide a pathway for designing novel NADES formulations tailored for specific industries, including their use as additives in anti-ice coatings.